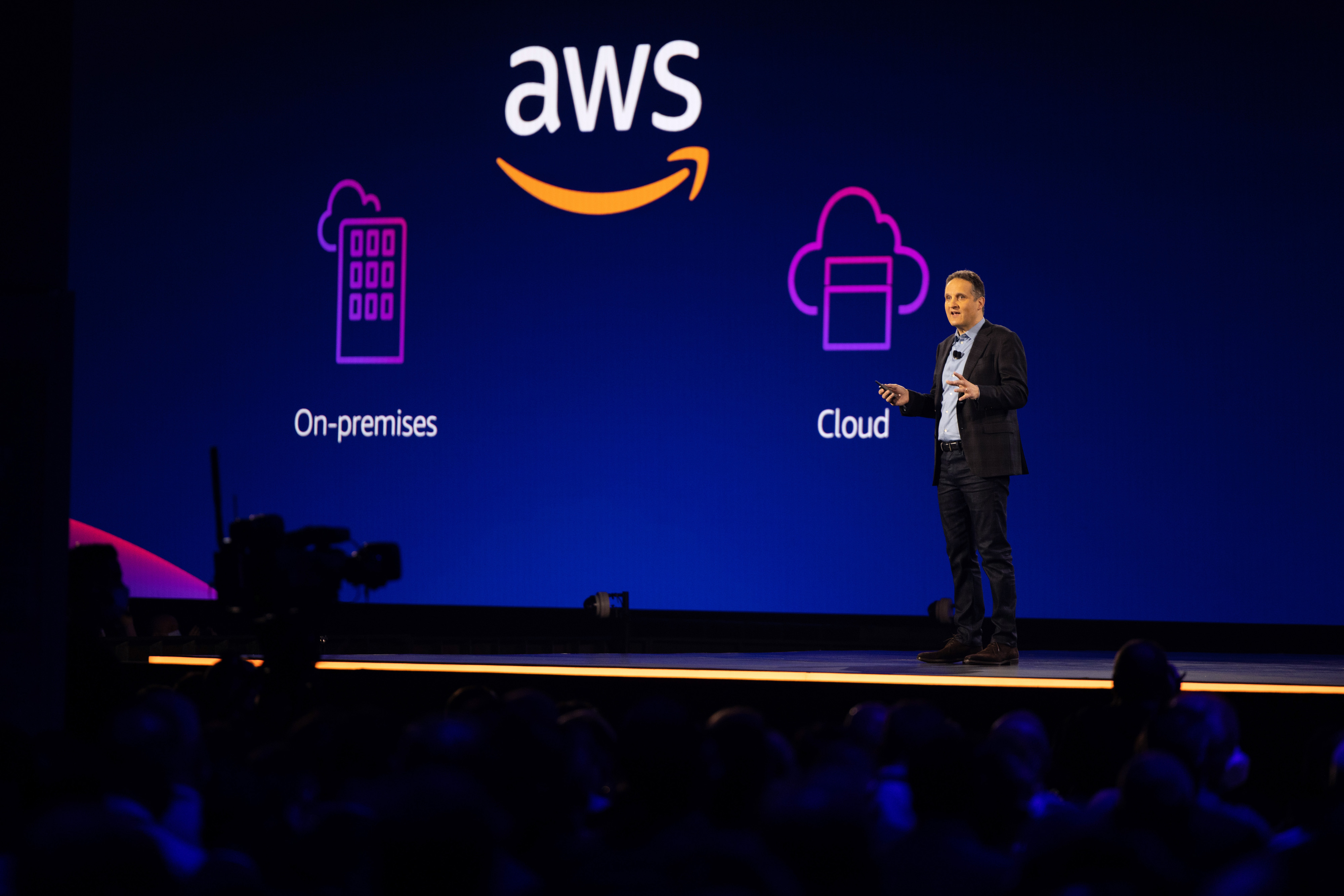
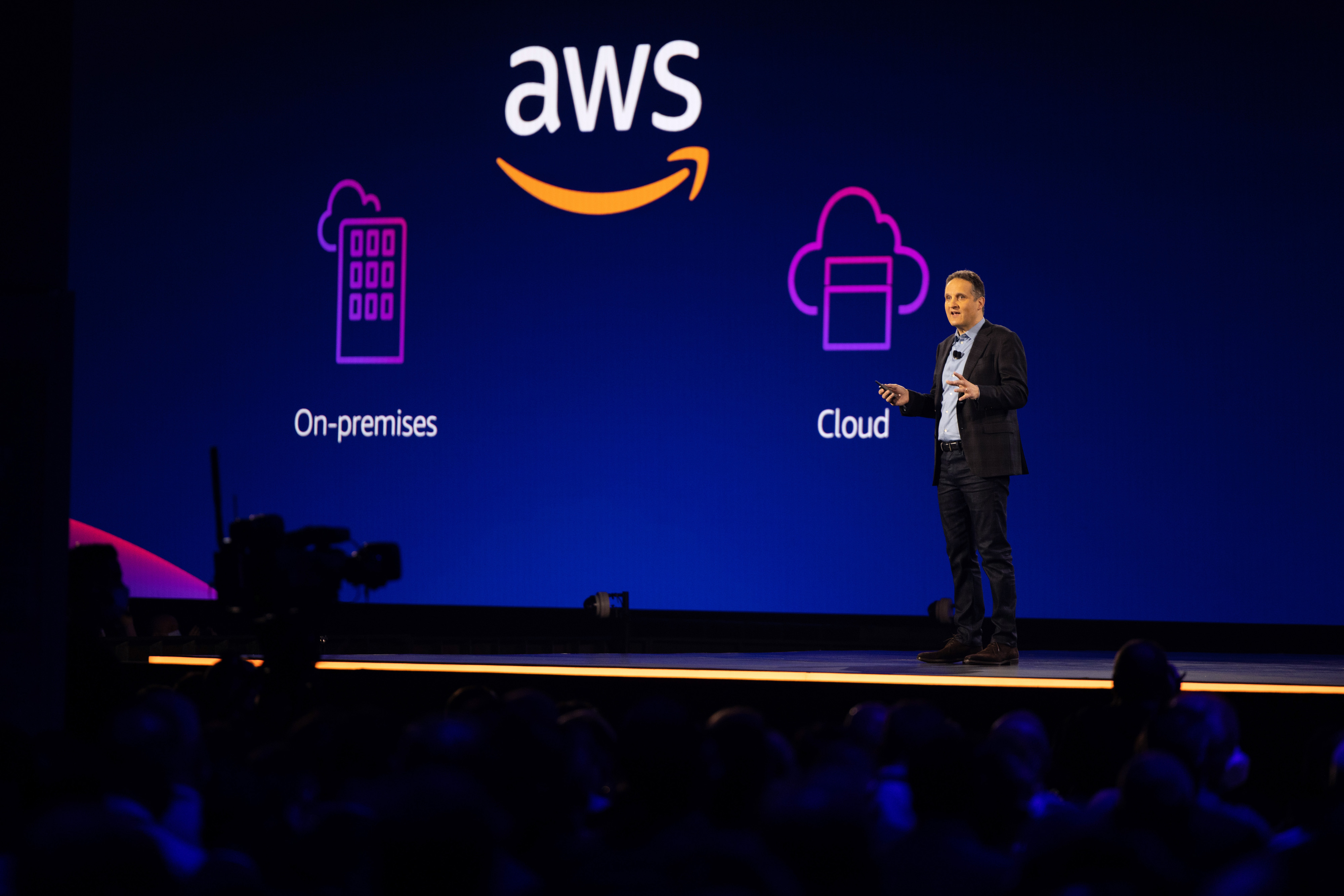
One reason Adam Selipsky was appointed chief executive of Amazon Web Services Inc. earlier this year was his extensive experience at AWS — but it’s a good bet that his tenure heading Tableau Software Inc. before and after its acquisition by Salesforce.com Inc. was a key factor as well.
After all, in some ways the cloud is all about the data and how to analyze it, which was Tableau’s specialty. In this third of a four-part interview with me and Wikibon Chief Analyst Dave Vellante ahead of the company’s re:Invent conference running this week in Las Vegas, Selipsky (pictured) dug into the kinds of services needed to deal with the continuing data deluge.
“At the end of the day, it is about that end-to-end capability set and that end-to-end journey that data has to traverse,” Selipsky says. “Machine learning capabilities will make all of that smarter over time. If you look forward a few years — I don’t think it’ll take 10 — our services will be helping you to make really intelligent choices, or will make those choices for you and you can approve them.”
You can get the big picture from Selipsky’s entire interview here, and don’t miss the other installments of the full interview on SiliconANGLE, with one more to come. Also, check out wall-to-wall coverage of re:Invent by theCUBE, SiliconANGLE Media’s livestreaming studio, and SiliconANGLE all this week and beyond for exclusive interviews with AWS executives and others in the AWS ecosystem. If you’re at re:Invent, stop by theCUBE’s studio in the exhibit hall.
This interview was edited for clarity. (* Disclosure: SiliconANGLE and theCUBE are paid media partners at AWS re:Invent. AWS and other sponsors have no editorial control over content on SiliconANGLE or theCUBE.)
Furrier: What’s the role of data in an artificial intelligence-driven specialization for individual vertical industries?
Data and how data is managed is a huge issue and a huge opportunity in the world today. That is only going to accelerate. I’ve seen projections saying that in the three years ending in 2024, that there’ll be more data created in the world than in the prior 30.
It’s a huge problem for those who don’t know how to deal with that data. I like the expression, “The safest place to hide is in plain sight.” If you can’t find the truth in your data because you are overwhelmed by the volume of your data, then you have a problem.
But it’s also an incredibly exciting opportunity for organizations that do put the scaffolding and the infrastructure and the capability in place to deal with that data. And you’ll see entirely new industries and entirely new capabilities within existing companies created. The way we’re thinking about the future of data and the future of capabilities that people need from us is, first and foremost, that point solutions are not the answer.
Data goes on a journey. It’s not a snapshot, and so data comes in from somewhere and it might be from an industrial sensor, or it might be from web logs, might be metadata on photos that are stored in the cloud. So data comes in and it has to land somewhere. It’ll often land in a data lake, you got to have data lakes. It might go from there to a database, or it might have come into a database and then gone to the data lake, and then you’ve got to have all sorts of capabilities to query that data.
Furrier: What kinds of capabilities?
There’s not one right answer as to “How do you query that data?” You’ve got relational databases, you’ve got nonrelational databases, you’ve got data warehouses, and all of those are the right tools for certain jobs. And so customers need us to have a variety of those tools and that’s why we have
15 different databases. And then we’ve got to have analytics, the tools to analyze and query that, and of course you want that to expand to your business users, not just your developers or IT staffs. So we’ve got things like QuickSight, for BI and visualization and to collaborate and share.
You really have to look at the end-to-end journey of data. Today we have by far the most complete and powerful set of end-to-end services for the journey on which that data has to go. And then it’s important that we begin, and you see this happening now in AWS, to infuse machine learning and AI into that. You’ve got all these ML capabilities, which will only make these data lakes, databases, analytics services smarter, more able to do predictive modeling, more able to make choices about which service is right to query a certain data set.
Customers ask AWS to have a first-party solution for a lot of those components, and then in some cases they also want to use third-party offerings and we make it really seamless for those to snap in. And we fully support those partners and whatever customers want. But at the end of the day, it is about that end-to-end capability set and that end-to-end journey that data has to traverse.
Furrier: What’s the role of machine learning at AWS?
ML capabilities will make all of that smarter over time. If you look forward a few years — I don’t think it’ll take 10 — our services will be helping you to make really intelligent choices, or will make those choices for you and you can approve them in terms of what the journey of that data looks like, and what are the tools that operate on that data, because we’ve got a lot of tools today.
An opportunity we have is to continue to make it even easier over time to know which tool to use and to know how to use it. As the cloud just becomes more and more ubiquitous and as it goes to tens of millions and hundreds of millions of practitioners who are interacting with the cloud, they’re not all going to be technical users. We want to make it really simple for people to interact with the cloud, even if they don’t know what an API is.
Vellante: The easier you make it to consume data, the more value customers are going to get, and we’ve seen a number of your customers, JP Morgan, Hello Fresh, Intuit, Roche, all of them, they’re bringing their own bucket, and they’re essentially democratizing access to the data. But there’s a real lack of standards to share data, govern data, enable self-service automation. How do you think about filling those gaps? Is it machine learning automation of the tooling, or what?
Data governance is an absolutely critical and central topic. Particularly in larger enterprises over the past decade, there’s been an explosion of data. There’s been a lot of tools that are really powerful for analyzing that data. And a lot of enterprises have been petrified of who might see that data and what they might do with it.
So a lot of them have locked it down. You end up actually not with democratized data, but rather with a few guardians of the data in a high tower and colored robes who then produce reports and send static reports out to lots of people. And if you want that static report to change, you put in a ticket and if you’re lucky, 15 weeks later you’ve got a column F on your static report if somebody’s decided that you’re actually allowed to see that data.
It’s because of a lack of governance. So what you’ve really seen is the whole segment, including AWS, really trying to provide governance capabilities. Basically if you provide companies with the ability to govern who can and cannot do various things with which data, it actually sets them free to provide access to that data. It’s a little bit counterintuitive, but it’s the ability to govern which gives them comfort to democratize.
AWS filled a lot of governance capabilities inside of our data lake capability, inside of Lake Formation. We’ve got services like Control Tower, which help you set up parameters. Part of governance is the physical location of data. We’ve given customers very strict control over where their data actually sits, and unlike some other services, if you put your data in Germany, if you put your data in Australia, if you put it in Oregon, it stays there. We’re not moving it. There’s not a global metadata service where we ship metadata around the planet, because data residency, whether it’s for national sovereignty or other reasons, is very important to our customers.
Furrier: How will enterprises take advantage of data governance capabilities?
I think it’s happening now. This is going to be a multiyear journey, as is everything in the cloud. But we’ve already built a lot of capabilities in this area, we’re just going to continue to iterate really quickly. It gets back to the organization, not the developer, the organization setting up a governance structure that makes sense for it. Some organizations will actually choose to be more restrictive about who gets access to what data and other organizations are going to be incredibly permissive. They’re going to say, let’s expose our data lake to everybody because I can’t predict what some associate product manager will go and dream up and start cross correlating different things out of my data lake. And she’s come up with some amazing insight.
But I think where we’re heading is to do things like having really robust and really flexible and fine-grade access controls. So you’ll be able to say, people in this country or people of this job class or people of this level of seniority in the organization have access to this type of data, or have access to this data source, or have access to this row and this column within this data source. Over time, you’ll just have more and more powerful capabilities in terms of being able to tag that data and in terms of understanding the lineage of that data, where it came from and where it’s going. Those governance tools, which will be decided on by the organization overall, will put this governance framework in place that hopefully makes sense for that organization.
I think most organizations will be quite permissive because of the innovation that can happen when you unlock that data. I think most organizations will choose to be permissive and just disable access to things that are incredibly sensitive. But then you will have developers who will be doing self-service analytics, who will be querying data lakes and databases, who will be doing so with drag-and-drop interfaces, who will be, in fact, applying machine learning without being machine learning experts. More and more, you’ll see us democratizing machine learning that becomes an analytics tool not for the professional practitioner.
Your early messaging with Amazon the early days was, “We do all the heavy lifting for you.” There’s a lot of heavy lifting going on in data, too. There’s a lack of capabilities, a lot of slowness, so it just feels like there’s a potential DevOps replay.
I think there is a good analogy there. Some of it is just continuing to build out the base-level capabilities. But looking ahead to the nontechnical practitioner and looking ahead to people who just want to ask questions about their data, and giving them very intuitive ways that jell with the way that the human mind already works, will be the key. And I think in some ways that is like making infrastructure code and the DevOps movement.
Tomorrow: Adam Selipsky reveals why AWS views designing its own chips is so important and digs into how he views the evolution of edge and hybrid cloud computing.
THANK YOU